Cybersecurity threats are evolving rapidly, and tech experts are not the only ones noticing. Even the general public is becoming more aware of the security issues that often make headlines.
Understanding these risks isn’t just for IT professionals anymore; it’s something we all need to grasp. The tricky part is figuring out who could be targeted, why these attacks happen, and when you might find yourself vulnerable.
Experts project that by 2025, cybercrime costs could skyrocket to $10.5 trillion, highlighting the growing audacity and sophistication of these attacks. In fact, it’s estimated that face spoofing attacks in identity verification processes are responsible for a large chunk of these security breaches, making it a major concern in today’s digital landscape.
So, what exactly is face spoofing?
It’s a method used to deceive biometric facial recognition systems by presenting false images, videos, or even masks to impersonate someone else.
These attacks are becoming more sophisticated, especially with the rise of deepfakes and image injections, which can create highly realistic, fake representations of individuals. This puts identity-based verification methods, such as those used for banking or unlocking devices (KYC), at risk.
In this blog, we’ll break down the ins and outs of face spoofing. You’ll learn about the methods hackers use, the potential impacts of identity spoofing, and, most importantly, the solutions available to protect yourself and your business from these attacks.
So, let’s get started!
The Impact of Facial Spoofing Methods
Now that we’ve explored what face spoofing is, it’s time to dig into the methods attackers use and their potential impacts. Fraudsters could use a range of tactics to get the better of identity verification systems, including:
- Acquiring access to buildings with facial recognition systems, perhaps stealing important corporate data kept there (ID theft)
- Creating false identities to sign up for services and engage in various frauds (insurance fraud, iGaming fraud, etc.)
- Adopting somebody else’s identity (attacks involving impersonation)
- Avoiding KYC and screening processes, or more generally, avoiding system recognition (obfuscation attacks)
Facial spoofing, also referred to as presentation attacks, isn’t just about hacking into a system; it’s about the ripple effect that follows, affecting security, finances, and trust.
Having said that, we’ll discuss the various types of facial spoofing attacks and their consequences.
Types of Facial Spoofing Attacks
Attackers try to trick facial recognition systems in several ways, each with its own unique approach and level of sophistication. Let’s take a closer look at the most common forms of spoofing.
Photo Spoofing
One of the simplest methods is photo spoofing, where attackers use a static image of the person they’re impersonating to trick the facial recognition system. This might seem rudimentary, but it’s shockingly effective against systems not built with robust liveness detection.
Imagine someone holding up a printed photograph or showing a high-resolution digital image in front of a camera, convincing the system that it’s a real person.
While some newer systems are evolving, many still fall victim to this basic form of attack.
Video Replay Attacks
A more advanced approach is video replay attacks, where attackers use a recorded video of a legitimate user to bypass the system.
Unlike photo spoofing, the dynamic movement in the video can trick systems that rely on motion to verify identity.
For example, a hacker might capture a video of you blinking or nodding and then replay that video to impersonate you during a facial recognition process. Since the video mimics natural behavior, it can be pretty difficult for unsophisticated systems to detect these attacks.
3D Mask Attacks
Perhaps the most elaborate form of spoofing is the use of 3D mask attacks.
In these cases, attackers create realistic, three-dimensional masks that mimic a target’s facial features. These masks can even include skin texture and expressions, making them extremely difficult to distinguish from a real face.
Hackers have successfully used silicone and other materials to create such masks, allowing them to bypass even some of the more advanced facial recognition systems. Moreover, these types of attacks are rare but can be devastating when successful.
Consequences of Spoofing
Now, let’s discuss the consequences of these spoofing methods. Each attack can have far-reaching effects, from security breaches to financial losses, not to mention the growing mistrust in facial recognition technology.
Security Vulnerabilities
When a facial recognition system is successfully spoofed, it opens up significant security vulnerabilities. Hackers can gain unauthorized access to devices, bank accounts, and even sensitive data, putting personal and corporate security at risk.
For example, recent studies show that biometric spoofing attacks have increased by 50% since 2022. Many participants—about 70%—have expressed concerns about the security of biometric authentication methods. Another study indicated that more than 80% of tested fingerprint scanners could be compromised by spoofed fingerprints from materials like gelatin or silicone.
In 2002, a Japanese researcher named Tsutomu Matsumoto attempted to deceive a fingerprint sensor using a Gummy Bear candy. He created a replica of a fingerprint he lifted from a glass surface. Impressively, his homemade fake fingerprint managed to fool the sensor 80% of the time, demonstrating that even basic methods can sometimes bypass biometric security systems.
Financial Losses and Fraud
The financial toll of identity spoofing is staggering. It’s estimated that face spoofing contributes to the billions lost annually to fraud globally.
For instance, in 2019, a security breach at a biometric security firm called Suprema revealed the fingerprints and facial recognition data of more than a million individuals. The data, comprising 27.8 million records and totaling 23 gigabytes, was discovered in a publicly accessible database.
This shows that businesses not only lose money but also suffer reputational damage, and individuals affected by identity theft face a long road to recovery from fraud.
Loss of Trust in Biometric Systems
When attacks like these occur, they can lead to a widespread loss of trust in biometric systems. Imagine the frustration of relying on a technology that claims to be secure, only to find out it’s been easily fooled by a photograph or video.
As spoofing incidents grow, users may question the reliability of facial recognition systems. This ever-increasing skepticism could slow down the adoption of such technologies despite their convenience.
Combatting Facial Spoofing 101
Understanding how facial spoofing works is only part of the battle. The next step is to explore how we can defend against it. As attackers get smarter, so must our technology and strategies to protect our systems.
Understanding Spoofing Techniques
First, it’s essential to recognize how attackers are able to exploit weaknesses in current facial recognition systems.
These systems often fail because of vulnerabilities in their design. For example, many systems struggle to detect whether a face presented in front of the camera is truly “live.”
These systems can sometimes be tricked by static images or video replays, as they may not check for signs of liveness like eye movement or subtle facial muscle shifts. Older systems may also lack depth perception, making them particularly vulnerable to 3D mask attacks.
Additionally, some algorithms are not trained on a diverse enough dataset, leading to inaccurate detection and increasing the chances of successful spoofing.
Attackers can also exploit systems that do not integrate advanced anti-spoofing technologies like deep learning or AI-based liveness detection (which we’ll discuss in detail later!). These tools can analyze subtle details that a human eye might miss, such as light reflection on a real face versus a photo or the natural movement of skin.
Without such sophisticated defenses, even high-quality systems can fall prey to spoofing.
Regulatory and Compliance Considerations
There’s growing global awareness around the importance of regulations focusing on biometric security and anti-spoofing methods.
For instance, the General Data Protection Regulation (GDPR) in Europe requires companies to safeguard biometric data, imposing hefty penalties for breaches. This includes ensuring systems are secure from identity spoofing threats.
In the U.S., states like Illinois have introduced specific laws like the Biometric Information Privacy Act (BIPA), which mandates that businesses obtain explicit consent before collecting biometric data and implement strict security measures. Non-compliance with these regulations can result in legal consequences, including fines.
In 2020, the Patel v. Facebook, Inc. class action lawsuit concluded with Facebook agreeing to a $650 million settlement. This settlement resolved allegations that the company collected user biometric data without consent and marked one of the largest consumer privacy settlements in U.S. history.
These regulations push companies to adopt advanced anti-spoofing methods and keep them accountable for the safety of biometric data.
On an international scale, the ISO/IEC 30107-3 standard outlines Presentation Attack Detection (PAD) requirements, which guide companies in identifying and preventing spoofing attack attempts. This standard provides a global benchmark for evaluating whether facial recognition systems are equipped to defend against attacks like deep fakes and image injections.
By enforcing these frameworks, regulators ensure companies remain proactive in combating face spoofing. And as the technology behind biometric authentication systems evolves, we can expect to see even more robust legal protections on the horizon.
Robust Anti-Facial Spoofing Techniques
Several innovative techniques have been developed to strengthen facial recognition systems against attacks in recent years. From verifying a user’s presence to continuous monitoring, these technologies are shaping the future of biometric security.
Let’s dive into some of the most popular anti-spoofing techniques available today.
1. Liveness Detection
One of the most powerful ways to prevent face spoofing is through liveness detection, which ensures that the person in front of the camera is a real, live individual, not a photo, video, or mask.
Liveness detection typically involves motion detection, where the system prompts users to perform actions such as blinking, turning their heads, or smiling. These movements are difficult to replicate using photos or videos, making it harder for attackers to bypass the system.
Another effective method is physiological checks. For instance, modern systems can detect subtle cues such as changes in skin texture, lighting reflections on the face, or even the blood flow patterns under the skin—something that’s nearly impossible to mimic with a fake image or mask.
Apple’s Face ID employs similar active liveness detection technology. The groundbreaking technology has largely replaced the iPhone’s Touch ID, providing an additional layer of security for Apple devices. The dual-layer security of Face ID combined with a passcode offers greater protection than traditional facial recognition using a basic 2D front-facing camera.
Apple incorporates a TrueDepth camera in every iPhone, which utilizes an infrared camera system to scan the user’s face and generate a depth map of up to 30,000 invisible dots.
This face-scanning technology focuses on the eyes, nose, and mouth and employs liveness detection to ensure the user’s eyes are open and looking at the device. If the user is lying down, squinting, or has their eyes closed, Face ID will not recognize them.
Apple securely stores a mathematical representation of your face locally on your device. Importantly, this data is never backed up to the cloud, minimizing the risk of theft or breach of your biometric information.
2. Multi-Factor Authentication (MFA)
Relying solely on facial recognition isn’t always enough, which is why many security systems are turning to Multi-Factor Authentication (MFA).
MFA enhances security by requiring users to provide multiple pieces of evidence before granting access, making spoofing far more challenging. For example, financial services apps often combine facial recognition with a second factor, like a one-time password (OTP) sent to the user’s phone or a fingerprint scan.
This way, even if an attacker manages to bypass the facial recognition system using a deepfake or identity spoofing method, they would still need to overcome the secondary verification step.
A real-world example of this can be seen in banking apps like HSBC’s mobile app, which combines facial recognition with a fingerprint scan or voice recognition to prevent unauthorized access. This multi-layered approach adds an extra security checkpoint, significantly reducing the risk of successful spoofing attacks.
3. Continuous Monitoring and Adaptation
Continuous monitoring involves analyzing user behavior in real-time to detect anomalies that could indicate a spoofing attempt. This could include tracking how users interact with the system, such as how they move their mouse or type on a keyboard.
If the system detects unusual behavior—such as a sudden change in typing speed or mouse movement—it may trigger an additional verification step to ensure the user is legitimate.
Companies like BehavioSec—which has now been acquired by a global data and analytics platform called LexisNexis Risk Solutions—have already integrated this kind of real-time behavior analysis into their fraud detection platforms. By analyzing things like typing patterns, how users hold their phones, or even how they walk, these systems can catch potential identity fraud attempts early.
Continuous monitoring protects against face spoofing and adds another layer of security for sensitive transactions.
Emerging Technologies in Face Spoofing Prevention
The future of facial recognition security lies in embracing cutting-edge tools and methodologies that can outpace even the most sophisticated attacks.
Starting with,
AI and Machine Learning Applications
Artificial intelligence (AI) and machine learning (ML) have already revolutionized how we detect and prevent face spoofing. These technologies are designed to learn, adapt, and improve over time, making them ideal for spotting even the subtlest signs of fraudulent activity.
For example, AI face recognition can differentiate between real faces and fake ones by analyzing minute details such as how light reflects off the skin or the tiny movements of facial muscles that occur even when we’re sitting still. These systems get smarter over time, learning from each interaction to improve their detection rates.
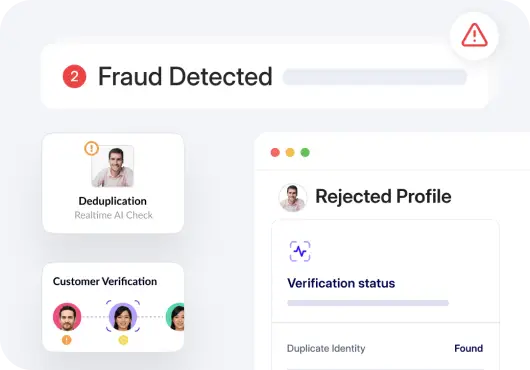
Use HyperVerge’s identity verification solution to analyze large data sets to detect patterns invisible to human analysts, enabling proactive fraud detection and prevention
HyperVerge is a digital identity verification platform that enables businesses to streamline their workflows with minimal or, in some cases, no coding required.
HyperVerge offers a powerful face recognition API that includes advanced AI-driven features such as:
- Deepfake detection
- Face de-duplication
- Forgery checks
- Biometric verification
- Liveness checks
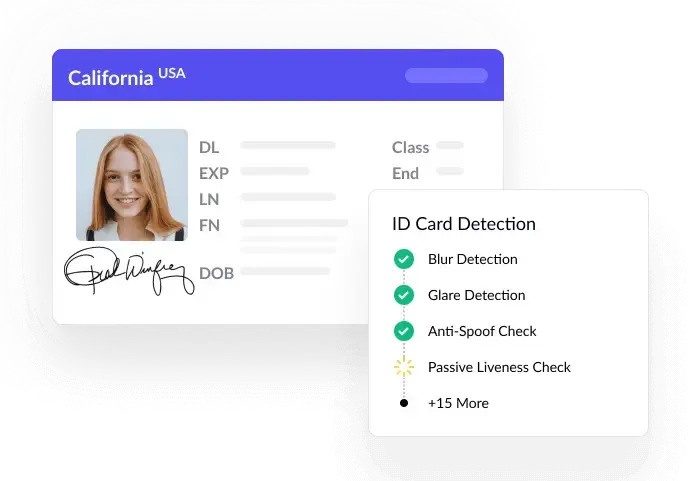
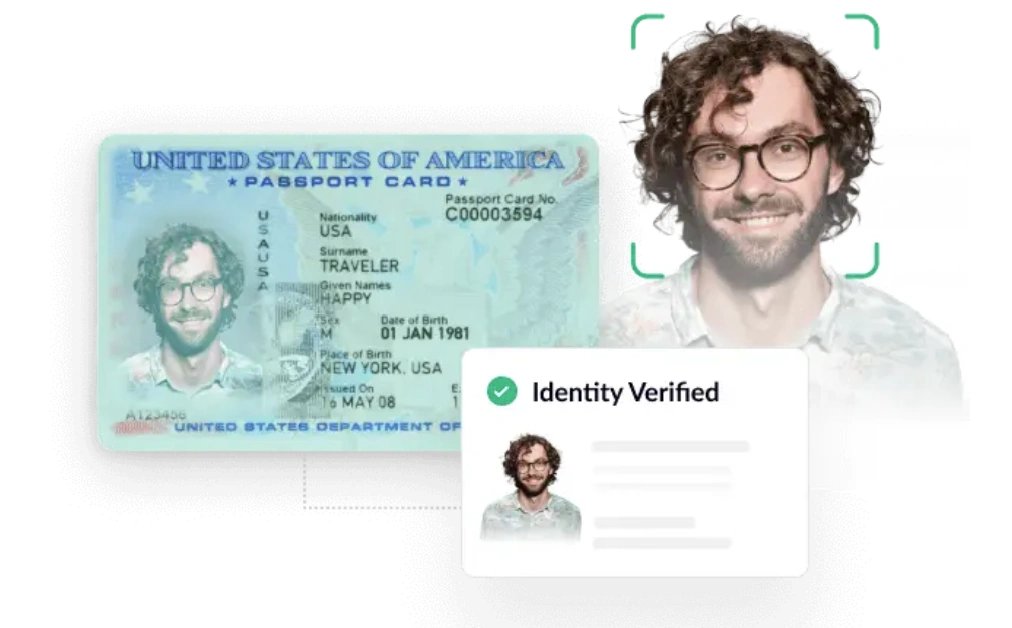
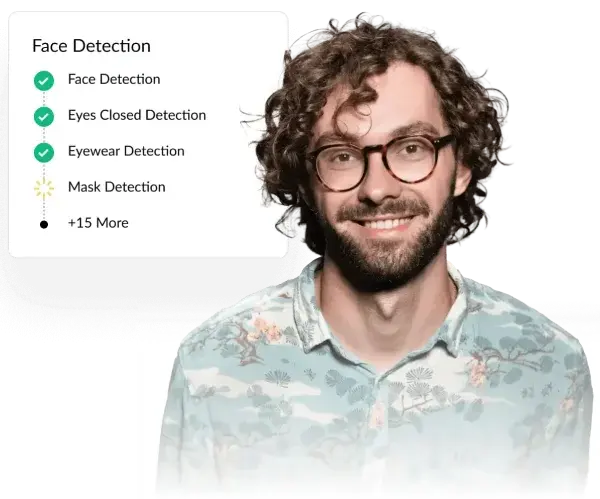
Ensure secure and accurate identity verification on different facial variations
Our AI models have been extensively trained on different facial variations, ensuring highly accurate results across different races, ages, and genders.
Powered by 13 years of AI development, HyperVerge’s face verification solution delivers effective and precise ID verification with an auto-approval rate exceeding 95% and can authenticate faces in just 0.2 seconds. The accuracy of HyperVerge’s passive liveness detection method has been certified by iBeta.
Additionally, our platform can reduce document collection time by up to 5 minutes, lower manual verification from 100% to 30% of customer applications, decrease customer drop-off rates by approximately 50%, and cut account activation turnaround time from hours to mere seconds.
Another fascinating AI application is deep learning neural networks. These networks can analyze thousands of facial images and videos to detect even the most advanced forms of spoofing. AI can pick up on unusual patterns in pixel movement or inconsistencies in how a person’s face looks when viewed from different angles—things that human eyes or older algorithms would struggle to catch.
Future Trends in Facial Recognition Security
As we enter a new era in technology, the field of biometrics is evolving rapidly. Traditional identity verification mechanisms are being refined, and new modalities are emerging. According to a recent report, the market for next-generation biometric technology is projected to reach USD 94.23 billion by 2028.
While AI and machine learning are currently leading the charge in preventing face spoofing, new technologies and trends are emerging that could push facial recognition security even further in the years to come.
Let’s take a look at some of them:
1. Lie Detection and Age Verification
Several countries have developed lie-detection programs that utilize facial recognition to interpret truthfulness based on a person’s expressions. This technology enables investigators to assess whether a suspect is being truthful.
The European Union has funded an initiative to create a virtual lie detector test, also known as the smart ‘deception detection’ system, that travelers to the EU could take from home using a live webcam. This technology analyzes facial expressions to gauge honesty, similar to how age verification functions in online applications.
2. Facial Recognition with Masks
The COVID-19 pandemic has posed challenges for facial recognition due to increased mask-wearing. However, technological advancements have led to impressive solutions, with some systems achieving 99% accuracy in recognizing masked faces.
For instance, NEC Corp. has developed a face recognition system that can identify individuals in just one second, first assessing mask status before focusing on visible facial features.
This technology is currently being used in various settings across the U.S. and Europe, including restaurants, hotels, and airports, to ensure compliance with health protocols.
3. Privacy Enhancing Technologies (PETs)
As privacy concerns grow, we may also see advancements in privacy-enhancing technologies. PETs can also:
- Minimize the use of personal data and maximize data security
- Allow organizations to work together on data analysis without sharing copies of the data
- Enable greater accountability through audit
- Provide access to data that might otherwise be restricted due to privacy, national security, or commercial sensitivity
These would allow users to authenticate their identity without sharing sensitive facial data, using cryptographic techniques like homomorphic encryption to keep biometric information secure during verification.
Combat Fraud With HyperVerge
Liveness detection technologies are set to become increasingly prevalent in the coming years. By combining deep learning and artificial intelligence, businesses can enhance facial anti-spoofing solutions.
HyperVerge is committed to pushing the boundaries of what’s possible, continuously benchmarking the performance of our AI-powered facial recognition and deep fake detection systems. Our comprehensive range of advanced identity verification solutions includes facial recognition, liveness detection, document identification, and more.
So, why wait? Sign up for free today to prevent and detect fraud as your business grows, ensuring your peace of mind.
FAQs
1. What is face spoofing?
Face spoofing is the act of deceiving facial recognition systems by using fake representations, such as photos or masks, to impersonate someone.
2. Can facial recognition systems be spoofed?
Yes, facial recognition systems can be spoofed, especially if they lack advanced features like liveness detection.
3. Which hybrid algorithm is used for face spoof detection?
Hybrid algorithms for face spoof detection often combine multiple techniques, such as machine learning, deep learning, and image processing. These methods might integrate features like texture analysis, motion detection, and biometric analysis to improve detection accuracy and reduce false positives.
4. What is the success rate of facial recognition?
Generally, modern facial recognition systems can achieve accuracy rates as high as 99.97% on standard assessments like NIST’s Facial Recognition Vendor Test (FRVT). However, the performance may decrease under challenging conditions like poor lighting or occlusions.